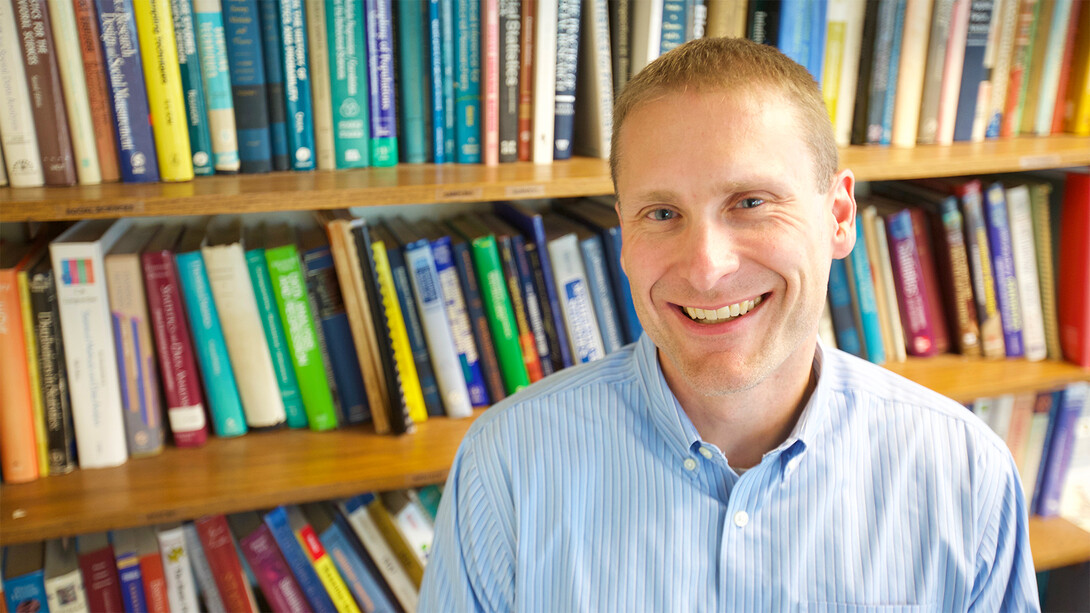
One bad apple can spoil the whole bunch. The truth of that adage may frustrate orchard owners and apple fiends, but statistician Chris Bilder can appreciate it.
That’s because Bilder knows the principle, exemplified by a statistical procedure called group testing, can help clinics and blood banks reduce the time and money spent screening for infections ranging from HIV to West Nile.
With the support of a $1.1 million grant from the National Institutes of Health, Bilder is leading efforts to refine and expand the use of group testing, also known as pooled testing. First proposed as a way to streamline screening for syphilis during World War II, the procedure aims to minimize the number of tests needed to identify infections in blood and urine samples.
An Iowa-based laboratory that tests for sexually transmitted diseases – and is collaborating with Bilder on the project – estimated that group testing saved it more than $3 million from 2009 to 2014.
Rather than testing a sample from each person, the procedure entails placing multiple samples into a pool and testing that first. If a pool of 100 people tests negative for a disease, all 100 can be declared free of infection – reducing the overall number of tests by 99. If that pool instead comes back positive, it might be divided into 10 groups of 10 that are tested again. And if even three of those 10 groups are positive, clinicians can then individually test the 30 samples in those groups – still cutting the total number of tests by 59.
“As long as the disease that you’re testing for has a low prevalence, you can really reduce the number of tests and associated costs needed to screen,” said Bilder, professor of statistics at the University of Nebraska-Lincoln.
But questions naturally arise about how to optimize the size of pools, Bilder said. If one large pool comes out negative, a clinic will save more tests than if it had initially split those samples into several smaller pools. Yet if that large pool turns up positive, the clinic might spend more subsequent tests ferreting out the positive samples than if it had started small.
Bilder has spent years investigating such issues and advancing the use of group testing in clinical settings. Alongside colleagues from the University of South Carolina, Clemson University, the University of Iowa, and the Centers for Disease Control and Prevention, Bilder is now exploring how to optimize the procedure when employing state-of-the-art tests that can screen for multiple diseases at once.
The Nebraska Community Blood Bank and Minnesota-based Memorial Blood Centers are providing the research team with data that will help it develop new algorithms, Bilder said. Those algorithms, in turn, should eventually guide decision-making on group testing at those and other blood banks.
“With these (multi-disease screens), group testing is applied without necessarily taking into account the best way to use it,” he said. “What we’re doing is developing new algorithms and examining how well they perform through statistical techniques, such as deriving the expected number of tests and performing computer simulations. Then we can make recommendations … so that, before people actually put these algorithms into use, they know which ones will be the most efficient.”
The team will also investigate how group testing can affect screening accuracy, specifically the incidence of false positives and false negatives. The ultimate goal, Bilder said, is to ensure that group-testing procedures are at least as accurate as individual screening.
“If you have a disease, what’s the probability that the test is going to find it? With these group-testing procedures, some measures of accuracy can go down a little bit, which obviously is disappointing,” he said. “In particular, a false negative error is important from a public health perspective, because that person who’s actually positive is going to be spreading the disease.”
To address this, Bilder and his colleagues will further analyze state-collected data on the incidence of chlamydia and gonorrhea in Iowa, Washington, Oregon, Idaho and Alaska. By comparing the result of a given test with a person’s associated risk factors – such as having physical symptoms or engaging in unprotected sex – the team hopes to characterize which scenarios might warrant the retesting of negative results.
“This idea has been done before, but not taking into account these additional risk factors,” Bilder said. “How can we use this back-end screening to improve the accuracy of tests? That information needs to be discovered.”
Bilder and his colleagues will receive their funding from the National Institute of Allergy and Infectious Diseases under grant number R01-AI121351.
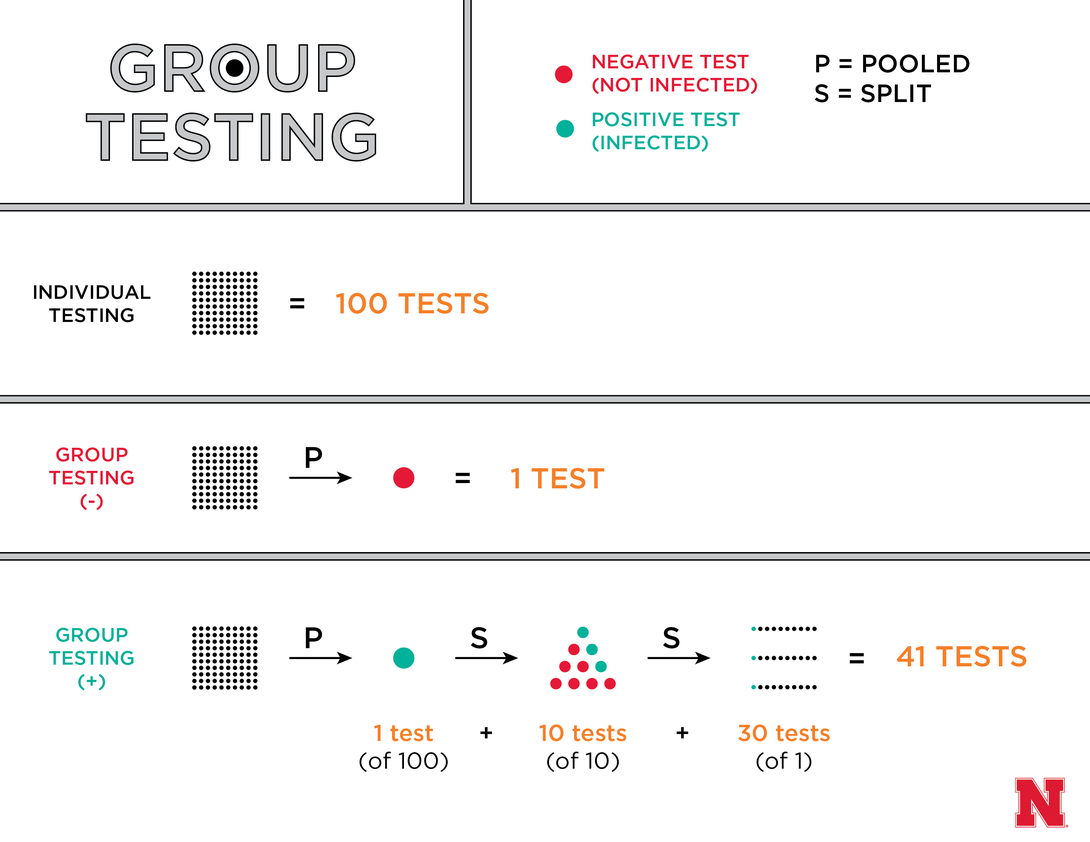